Generative AI: Redefining Data Science for the Future
Artificial intelligence (AI) has become a revolutionary force in the big data era part of data science, transforming industries and disrupting established practices. Generative AI is unique among AI subfields in that it can imitate human creativity to generate new content, including text, graphics, and even music. In addition to having an effect on the arts, this technology is dramatically altering the data science scene. We’ll examine how generative AI is changing the role of data science in this piece, as well as the consequences for the field’s future.
Top Companies' Adoption of Generative AI
Google's TensorFlow And Generative Adversarial Networks (GANs):
Leading AI research company Google has been aggressively investigating the uses of generative AI. The open-source machine learning framework TensorFlow from Google offers strong assistance in creating and implementing generative models. Notably, Generative Adversarial Networks (GANs) have been used by Google researchers to better natural language processing jobs, produce realistic photos, and improve healthcare diagnostics.
Facebook's PyTorch and Variational Autoencoders (VAEs):
Another well-known deep learning framework, PyTorch from Facebook, has also incorporated generative AI methods. Facebook uses generative models called variational autoencoders (VAEs) to produce high-quality photos, customize user experiences, and make content creation easier. Facebook’s generative AI research keeps expanding the realm of data science possibilities.
OpenAI's GPT Models:
OpenAI, a company well-known for its innovative artificial intelligence research, has released a set of Generative Pre-trained Transformer (GPT) models. With their extensive training on text data, these models are highly proficient at comprehending and producing natural language. Data scientists may simplify their workflows and open up new avenues for data analysis by using GPT models for tasks like text summarization, language translation, and even code generation.
How Data Scientists Are Empowered by Generative AI:
I - The Traditional Role of Data Science:
Let’s first look at the typical job of data scientists in order to comprehend the implications of generative AI. Large data sets have traditionally required the collection, purification, analysis, and interpretation by data scientists in order to derive useful insights. They use statistical methods, machine learning algorithms, and domain expertise to solve challenging issues, streamline workflows, and influence organizational decision-making. Their skillset encompasses recognizing trends, forecasting outcomes, and unearthing implicit connections concealed within the information.
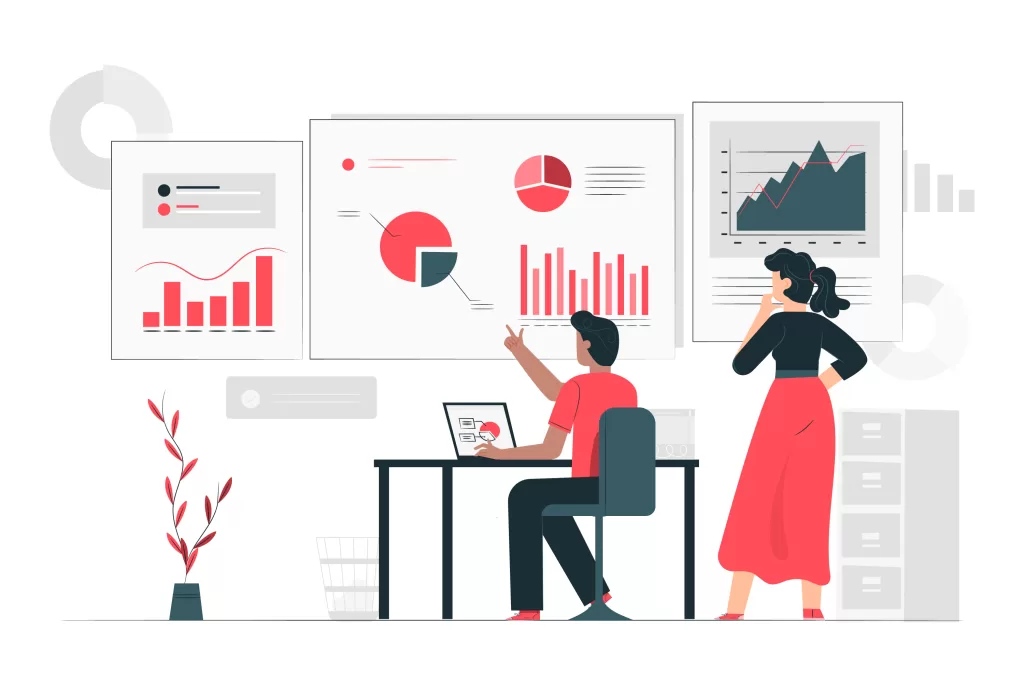
II - The Emergence of Generative AI:
A paradigm shift in the field of artificial intelligence is represented by generative AI. Generative AI models may create fresh data samples that are identical to genuine ones, in contrast to standard AI systems that are created for certain tasks like language translation or image categorization. Driven by deep learning methods like as variational autoencoders (VAEs) and generative adversarial networks (GANs), these models have proven to be remarkably adept at producing realistic text, images, audio, and even video.
III - Impact on Data Generation and Augmentation:
Data augmentation and generation is one of the main areas where generative AI is transforming the function of data scientists. In the past, data scientists trained machine learning models using pre-existing datasets. But getting tagged data can be expensive, time-consuming, and often unfeasible—particularly in fields with sensitive or sparse data. In order to overcome this difficulty, generative AI creates fresh data samples, hence increasing the size of the dataset and improving the variety of training examples. As a result, even with sparse labeled data, data scientists may create models that are more reliable and broadly applicable.
IV - Enhancing Data Exploration and Visualization:
Additionally, generative AI opens up new possibilities for data presentation and exploration. Data scientists can obtain deeper insights into underlying patterns and structures by using generative models to produce lifelike simulations or visualizations of complex datasets. Data scientists can discover outliers, investigate hypothetical scenarios, and gain a deeper understanding of the properties of their datasets by creating synthetic data representations. Making better informed decisions and formulating hypotheses is made easier with the help of this interactive data analysis method.
V - Automating Repetitive Tasks:
The automation of tedious operations is another important way that generative AI is changing the role of data scientists. In the data science workflow, features engineering, model tweaking, and data pretreatment are crucial yet time-consuming steps. By automatically creating synthetic features, enhancing training data, and optimizing hyperparameters, generative AI can expedite these procedures. This increases the productivity and efficiency of data scientists by freeing them up to concentrate on higher-level tasks like problem formulation, model interpretation, and domain expertise.
VII - Ethical and Bias Considerations:
Although generative AI has many advantages, data scientists need to address the ethical and prejudice issues it brings up. It is possible for generative models to reinforce or magnify preexisting biases in the training set, which could have unexpected repercussions in subsequent uses. In order to maintain fairness and transparency in AI-driven decision-making processes, data scientists must carefully consider the ethical implications of utilizing generative AI, eliminate biases through varied and representative training data, and put safeguards in place.
VII - Future Directions and Challenges:
It is probable that the position of data scientists will experience additional changes as generative AI develops. To properly leverage generative models, data scientists will need to adjust to new tools, approaches, and methodologies. The interdisciplinary cooperation of data scientists, machine learning engineers, domain specialists, and ethicists will be crucial in tackling intricate societal issues and optimizing the possible advantages of generative artificial intelligence. Further investigation is required to examine the ethical ramifications of AI-generated material across a range of fields and to create more reliable and understandable generative models.
Professionals may now upskill themselves with the recently released “Generative AI for Data Scientists Specialization” course from IBM and Coursera.
Information science will without a doubt be all the more profoundly influenced by generative man-made intelligence as it creates and develops. Generative models can possibly fundamentally expand the efficiency and adequacy of information researchers worldwide via computerizing tedious tasks and uncovering new bits of knowledge. Information researchers can stay on the ball and open up new roads for AI and information examination by embracing this game-evolving innovation.
Conclusion:
To sum up, generative AI is transforming data science by empowering researchers to create artificial intelligence (AI)-generated data, improve data exploration and visualization, automate tedious operations, and tackle intricate issues related to bias and ethics in AI. Although generative AI is becoming widely used, this opens up new possibilities but also brings with it difficulties and ethical issues that need to be carefully considered. Data scientists may fully utilize generative AI to spur innovation, expand knowledge, and improve society in the digital age by adopting it responsibly and ethically.